Data analysis: New models for faster and more accurate forecasts
Whether during a global pandemic, in retail, or in financial markets: In most cases, the more data you have to work with, the better. Processing large amounts of data can be challenging, however, because as data increases in complexity and volume, it becomes more and more complicated and time-consuming to process. Researcher Gregor Kastner from WU’s Institute for Statistics and Mathematics is currently working on a project funded by the FWF Austrian Science Fund with the goal of allowing large amounts of data to be processed faster and more easily.
Digitalization makes it possible to collect large amounts of data over extended periods of time, in so-called time series. The amount of data being collected is constantly increasing, be it in retail marketing, in fighting pandemics, in monitoring financial markets, or for use in economic analyses in research. According to Gregor Kastner, “We now have the technology to collect huge amounts of data and monitor many developments over long periods of time, for example the movements of financial markets, population developments, retail sales revenues, or the number of infections during a pandemic. But of course, the complexity of the information contained in this data increases with the amount of data. We are faced with the challenge of analyzing these often enormous amounts of data as rapidly as possible. Even with modern cloud computing solutions, however, it can take hours or even days to calculate modern statistical models – time we often just don’t have.” To solve this problem, Kastner is working in cooperation with an interdisciplinary team to develop algorithms and software that will deliver reliable results even faster.
Reducing complexity for more accurate forecasts
In the models, the researcher and his team focus only on the data required for analysis. “Simplification” is the key word here. “From a statistical perspective, the highly complex time series with huge amounts of data generated by the long-term monitoring of stock markets, population developments, etc. present us with a high-dimensional problem,” explains the statistician. “We reduce this high-dimensional statistical data space into a lower dimensional space by determining what the individual time series have in common and which information we don’t need to include in the analysis because it isn’t important for answering our questions.” A model that Kastner was involved in developing extracts the common dynamics of these time series to simplify the analysis: a factor stochastic volatility model that maps high-dimensional spaces into smaller, lower dimensional spaces. In addition, the researchers take a Bayesian approach, using so-called “shrinkage priors” to reduce the effects of superfluous parameters to close to zero. “This helps reduce unnecessary complexity in the model, which on the one hand saves time, while also improving the accuracy of forecasts of future developments,” says Kastner.
80,000 downloads: A further step towards a digital future
The new algorithms developed by Kastner are available for use in the open source, publically accessible statistics software R. This allows other researchers to be able to analyze large volumes of data more easily and to reach their own conclusions and predictions. “My software tools can help users master some of the processing challenges presented by the digital age,” says Kastner. Demand for Kastner’s software is high: The software packages have been downloaded over 80,000 times from RStudio’s CRAN mirror alone. The first application areas are also already being found, especially stock and exchange rates, macroeconomic data analyses like the economic cycle, and in monitoring product price developments.
The research project, headed by Gregor Kastner, is being carried out in cooperation with the University of Salzburg, TU Wien, and the Austrian Institute of Economic Research, and was awarded a €2 million grant from the FWF Austrian Science Fund in 2018 for interdisciplinary, cross-institutional Young Independent Researcher Groups.
Gregor Kastner
Gregor Kastner has been a researcher at WU’s Institute for Statistics and Mathematics since 2011. A native of Upper Austria, he has degrees in mathematics and information management as well as teaching degrees in information technology, mathematics, and physical education. In 2014, Kastner earned a doctorate in technical mathematics from the JKU Linz, graduating under the auspices of the Federal President of the Republic of Austria (sub auspiciis praesidentis rei publicae). He has gained international experience during a one-year stay at ETH Zurich and as a visiting scholar at the University of Chicago Booth School of Business (USA) and the Jiangxi University of Finance and Economics (China). Gregor Kaster’s research interests include Bayesian statistics and econometrics, computational statistics, and the mathematical modelling of uncertainty. He often cooperates on an interdisciplinary level with researchers in the fields of economics, agricultural economics, socioeconomics, biology, and information technology. His dissertation, entitled “Efficient Bayesian Inference for Univariate and High-Dimensional Stochastic Volatility Models,” won the Best Dissertation Award from the Austrian Statistical Association in 2015. Kastner has published successfully in internationally renowned journals, including the Journal of Econometrics, the Journal of Applied Econometrics, the Journal of Computational and Graphical Statistics, and the Journal of Statistical Software. He is a board member of the European Seminar on Bayesian Econometrics (ESOBE) and the Bayesian Young Statisticians Meeting (BAYSM), assistant editor of the Journal of Statistical Software, and associate editor of the ISBA Bulletin. Kastner has also been involved in organizing numerous international conferences and acts as a reviewer for leading journals in the fields of statistics and econometrics on a regular basis.
The study: Hosszejni, Darjus & Kastner, Gregor. (2019). Modeling Univariate and Multivariate Stochastic Volatility in R with stochvol and factorstochvol.
Additional papers
Software:
https://cran.r-project.org/package=factorstochvol
https://cran.r-project.org/package=stochvol
Contact:
Anna Maria Schwendinger
PR Manager
Tel: + 43-1-31336-5478
Email: anna.schwendinger@wu.ac.at
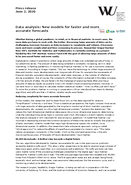